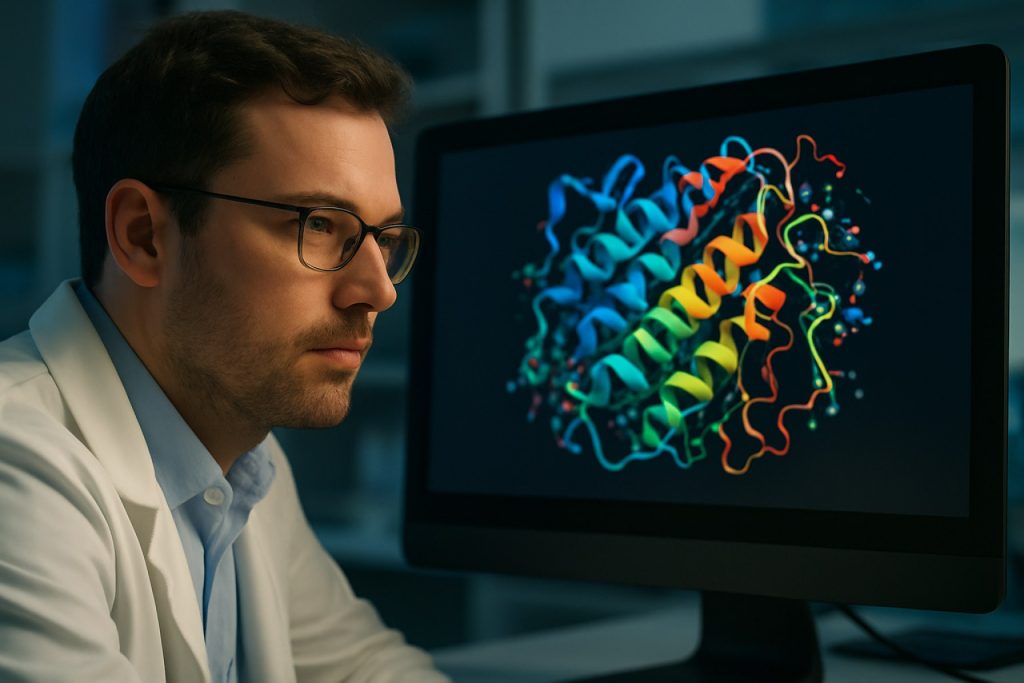
How the AlphaFold Algorithm is Transforming Biology: Unveiling the Secrets of Protein Folding and Accelerating Scientific Discovery (2025)
- Introduction to AlphaFold: Origins and Breakthroughs
- The Science Behind Protein Folding
- DeepMind’s Approach: How AlphaFold Works
- Key Achievements and Milestones
- Comparative Analysis: AlphaFold vs. Traditional Methods
- Applications in Drug Discovery and Biomedical Research
- Open-Source Impact and Community Collaboration
- Limitations, Challenges, and Ongoing Research
- Market and Public Interest: Growth and Forecasts
- Future Outlook: The Next Frontier in Computational Biology
- Sources & References
Introduction to AlphaFold: Origins and Breakthroughs
AlphaFold, developed by DeepMind, a subsidiary of Alphabet Inc., represents a transformative leap in computational biology. The algorithm was first introduced in 2018, but its most significant breakthrough came in 2020, when AlphaFold2 demonstrated unprecedented accuracy in predicting protein structures at the 14th Critical Assessment of Structure Prediction (CASP14) competition. This achievement marked a pivotal moment, as protein folding had been a grand challenge in biology for over 50 years. AlphaFold’s success was recognized by the scientific community as a solution to a problem that had stymied researchers for decades.
The core innovation of AlphaFold lies in its use of deep learning techniques to predict the three-dimensional structures of proteins from their amino acid sequences. By leveraging vast datasets of known protein structures and sequences, AlphaFold’s neural networks learned to infer spatial relationships and folding patterns with remarkable precision. The release of AlphaFold2 in 2021 further improved accuracy, with predictions often rivaling experimental methods such as X-ray crystallography and cryo-electron microscopy.
In July 2021, DeepMind and the European Molecular Biology Laboratory’s European Bioinformatics Institute (EMBL-EBI) launched the AlphaFold Protein Structure Database, making hundreds of thousands of predicted protein structures freely available to the global scientific community. By 2023, this database had expanded to include over 200 million protein structures, covering nearly every known protein sequence cataloged in the UniProt database. This open-access resource has accelerated research in fields ranging from drug discovery to synthetic biology.
As of 2025, AlphaFold continues to shape the landscape of structural biology. Ongoing developments focus on improving the algorithm’s ability to predict protein complexes, membrane proteins, and the effects of mutations. The open-source release of AlphaFold’s code has spurred a wave of innovation, with researchers worldwide adapting and extending the algorithm for specialized applications. Major organizations such as National Institutes of Health and Royal Society of Chemistry have highlighted AlphaFold’s impact on biomedical research and education.
Looking ahead, the next few years are expected to bring further integration of AlphaFold into experimental workflows, enhanced prediction of protein-protein interactions, and the development of next-generation algorithms that build on AlphaFold’s foundation. The algorithm’s influence is poised to expand as it becomes an indispensable tool for understanding the molecular machinery of life.
The Science Behind Protein Folding
The AlphaFold algorithm, developed by DeepMind, represents a transformative advance in the science of protein folding. Since its landmark performance at the 14th Critical Assessment of protein Structure Prediction (CASP14) in 2020, AlphaFold has continued to evolve, with its impact accelerating into 2025 and beyond. The core scientific challenge addressed by AlphaFold is the prediction of a protein’s three-dimensional structure from its amino acid sequence—a problem that has stymied biologists for decades due to the astronomical number of possible conformations a protein chain can adopt.
AlphaFold’s approach leverages deep learning, specifically attention-based neural networks, to model the spatial relationships between amino acids. The algorithm is trained on vast datasets of known protein structures, primarily sourced from the Worldwide Protein Data Bank (wwPDB), which is a global repository of experimentally determined protein structures. By learning from these data, AlphaFold can infer the likely distances and angles between residues in a new sequence, assembling a highly accurate 3D model.
In 2021, DeepMind and the European Molecular Biology Laboratory’s European Bioinformatics Institute (EMBL-EBI) launched the AlphaFold Protein Structure Database, making hundreds of thousands of predicted structures freely available. By 2025, this database has expanded to cover nearly all catalogued proteins, including those from humans, plants, bacteria, and other organisms, providing an unprecedented resource for the life sciences community.
Recent years have seen the release of AlphaFold2 and subsequent refinements, with ongoing research focused on improving predictions for protein complexes, membrane proteins, and intrinsically disordered regions—areas where traditional methods and even early versions of AlphaFold struggled. The algorithm’s open-source release has spurred a wave of innovation, with academic and industry groups building on its architecture to tackle related challenges, such as predicting protein-ligand interactions and modeling the effects of mutations.
Looking ahead, the scientific outlook for AlphaFold and its successors is highly promising. The integration of experimental data, such as cryo-electron microscopy and mass spectrometry, is expected to further enhance prediction accuracy. Moreover, the algorithm’s ability to accelerate drug discovery, enzyme engineering, and synthetic biology is being actively explored by organizations including National Institutes of Health and World Health Organization. As computational power and biological datasets continue to grow, AlphaFold’s foundational role in unraveling the complexities of protein folding is set to deepen, shaping biomedical research for years to come.
DeepMind’s Approach: How AlphaFold Works
AlphaFold, developed by DeepMind, represents a transformative leap in computational biology, specifically in the prediction of protein structures. The algorithm’s core innovation lies in its use of deep learning to predict the three-dimensional structure of proteins from their amino acid sequences, a challenge that has persisted in biology for decades. AlphaFold’s approach integrates advances in neural network architectures, attention mechanisms, and evolutionary data analysis, enabling it to achieve unprecedented accuracy in structure prediction.
The AlphaFold algorithm operates by leveraging large-scale multiple sequence alignments (MSAs) and structural templates, which are processed through a sophisticated neural network. This network is designed to model the spatial relationships between amino acids, predicting inter-residue distances and angles. The system iteratively refines its predictions, using a process akin to gradient descent, to converge on the most probable protein conformation. The latest version, AlphaFold2, introduced a novel architecture called the “Evoformer,” which efficiently captures both sequence and structural information, and a structure module that directly outputs atomic coordinates.
Since its public release, AlphaFold has had a profound impact on the scientific community. In 2021, DeepMind, in collaboration with the European Molecular Biology Laboratory’s European Bioinformatics Institute (EMBL-EBI), made the AlphaFold Protein Structure Database freely available, providing predicted structures for hundreds of thousands of proteins. By 2025, this database has expanded to cover nearly all known proteins, including those from humans, plants, bacteria, and other organisms, dramatically accelerating research in fields such as drug discovery, enzyme engineering, and disease understanding.
AlphaFold’s methodology continues to evolve. DeepMind and EMBL-EBI are actively updating the database and refining the algorithm to handle more complex protein assemblies, such as protein-protein interactions and multi-chain complexes. The open-source release of AlphaFold’s code has also spurred a wave of community-driven improvements and adaptations, with researchers worldwide integrating AlphaFold into their workflows and developing complementary tools.
Looking ahead, the next few years are expected to see further enhancements in AlphaFold’s capabilities, including improved modeling of protein dynamics, post-translational modifications, and interactions with small molecules. These advances are anticipated to deepen our understanding of biological processes and accelerate the development of novel therapeutics, solidifying AlphaFold’s role as a foundational tool in modern biology.
Key Achievements and Milestones
Since its introduction, the AlphaFold algorithm has marked a transformative era in computational biology, particularly in the field of protein structure prediction. Developed by DeepMind, a subsidiary of Alphabet Inc., AlphaFold’s most significant milestone came in 2021 when it demonstrated unprecedented accuracy in the 14th Critical Assessment of Structure Prediction (CASP14), outperforming all competitors and achieving results comparable to experimental methods. This breakthrough was widely recognized as a solution to the decades-old “protein folding problem.”
In 2022, DeepMind, in collaboration with the European Molecular Biology Laboratory’s European Bioinformatics Institute (EMBL-EBI), released the AlphaFold Protein Structure Database. This open-access resource initially contained over 350,000 predicted protein structures, including nearly all human proteins. By 2023, the database had expanded to cover over 200 million protein structures, representing nearly every known protein sequence cataloged in the UniProt database. This scale of coverage has enabled researchers worldwide to access high-quality structural predictions, accelerating discoveries in drug development, enzyme engineering, and disease research.
In 2024, AlphaFold’s impact continued to grow as its predictions were integrated into major biological research pipelines. The algorithm’s open-source code and model weights, released by DeepMind, have empowered the scientific community to adapt and extend the technology for specialized applications, such as modeling protein complexes and predicting the effects of mutations. Notably, AlphaFold’s predictions have been cited in thousands of peer-reviewed publications, underscoring its widespread adoption and influence.
Looking into 2025 and the coming years, AlphaFold’s trajectory is set to advance further. Ongoing collaborations between DeepMind, EMBL-EBI, and other leading research institutions are focused on refining the algorithm to handle more complex biological assemblies, such as multi-protein complexes and membrane proteins. Efforts are also underway to improve the accuracy of predictions for intrinsically disordered regions and to integrate AlphaFold with other computational and experimental methods for a more comprehensive understanding of protein function.
The outlook for AlphaFold remains highly promising. As the algorithm continues to evolve, it is expected to play a pivotal role in personalized medicine, synthetic biology, and the rapid response to emerging pathogens. The continued expansion of the AlphaFold Protein Structure Database and the development of next-generation algorithms will likely cement AlphaFold’s position as a cornerstone technology in the life sciences for years to come.
Comparative Analysis: AlphaFold vs. Traditional Methods
The advent of the AlphaFold algorithm has marked a transformative shift in the field of protein structure prediction, especially when compared to traditional experimental and computational methods. As of 2025, AlphaFold, developed by DeepMind—a subsidiary of Alphabet Inc.—continues to set new benchmarks in accuracy, speed, and accessibility for protein structure determination.
Traditional methods for elucidating protein structures, such as X-ray crystallography, nuclear magnetic resonance (NMR) spectroscopy, and cryo-electron microscopy (cryo-EM), have long been the gold standards. These techniques, while highly accurate, are resource-intensive, often requiring months or years of laborious experimentation, specialized equipment, and significant financial investment. For example, X-ray crystallography necessitates the crystallization of proteins, a process that is not always feasible, especially for membrane proteins or large complexes. NMR is limited by protein size, and cryo-EM, though increasingly powerful, still demands substantial computational and infrastructural resources.
AlphaFold’s approach, leveraging deep learning and vast protein sequence databases, has dramatically reduced the time and cost associated with protein structure prediction. Since its landmark performance in the 14th Critical Assessment of Structure Prediction (CASP14) in 2020, AlphaFold has been widely adopted by the scientific community. By 2025, the DeepMind AlphaFold Protein Structure Database, developed in partnership with the European Molecular Biology Laboratory’s European Bioinformatics Institute (EMBL-EBI), contains over 200 million predicted protein structures, covering nearly all known proteins catalogued in major sequence databases.
Comparative analyses published by leading research organizations demonstrate that AlphaFold achieves atomic-level accuracy for a significant proportion of proteins, rivaling experimental results in many cases. For instance, the Research Collaboratory for Structural Bioinformatics (RCSB) Protein Data Bank has reported that AlphaFold predictions often align closely with experimentally determined structures, especially for globular proteins. However, certain limitations remain: AlphaFold’s predictions are less reliable for intrinsically disordered regions, protein complexes, and proteins with rare folds not represented in training data.
Looking ahead, the integration of AlphaFold with experimental pipelines is expected to accelerate discovery in structural biology, drug design, and synthetic biology. Ongoing collaborations between DeepMind, EMBL-EBI, and other global research institutions are focused on improving predictions for protein-protein interactions and dynamic conformational states. As computational power and algorithmic sophistication continue to advance, AlphaFold and its successors are poised to further narrow the gap between in silico predictions and experimental validation, reshaping the landscape of molecular life sciences in the coming years.
Applications in Drug Discovery and Biomedical Research
The AlphaFold algorithm, developed by DeepMind, has rapidly transformed the landscape of drug discovery and biomedical research since its public release. As of 2025, AlphaFold’s ability to predict protein structures with high accuracy has been integrated into numerous research pipelines, accelerating the identification of drug targets and the understanding of disease mechanisms.
A major milestone was the release of the European Molecular Biology Laboratory’s European Bioinformatics Institute (EMBL-EBI) AlphaFold Protein Structure Database, which now contains predicted structures for over 200 million proteins. This resource, freely accessible to the global scientific community, has enabled researchers to investigate previously intractable proteins, including those from pathogens and rare diseases, thus broadening the scope of druggable targets.
In 2025, pharmaceutical companies and academic groups are leveraging AlphaFold to streamline the early stages of drug discovery. By providing accurate models of protein targets, AlphaFold reduces the need for time-consuming and costly experimental structure determination. This has led to a surge in structure-based drug design projects, particularly for proteins that were previously considered “undruggable” due to lack of structural data. For example, several collaborations between DeepMind, EMBL-EBI, and leading pharmaceutical firms have resulted in the identification of novel binding sites and the optimization of lead compounds for diseases such as cancer, neurodegeneration, and infectious diseases.
- Target Identification and Validation: AlphaFold’s predictions are being used to annotate protein function and to prioritize targets for therapeutic intervention, especially in genomics-driven drug discovery.
- Structure-Based Drug Design: Medicinal chemists are utilizing AlphaFold models to perform virtual screening, molecular docking, and rational drug design, significantly shortening the lead optimization cycle.
- Antibody and Vaccine Development: The algorithm’s capacity to model antigen-antibody interactions is aiding the design of next-generation biologics and vaccines, as seen in ongoing efforts against emerging infectious diseases.
Looking ahead, the integration of AlphaFold with other AI-driven tools and experimental methods is expected to further enhance its impact. Initiatives by organizations such as National Institutes of Health (NIH) and World Health Organization (WHO) are supporting the adoption of AlphaFold in global health research, with a focus on neglected diseases and pandemic preparedness. As the algorithm continues to evolve, its applications in drug discovery and biomedical research are poised to expand, driving innovation and collaboration across the life sciences.
Open-Source Impact and Community Collaboration
The open-source release of the AlphaFold algorithm by DeepMind in 2021 marked a transformative moment for computational biology, and its impact continues to expand in 2025. By making both the AlphaFold codebase and the predicted structures of hundreds of millions of proteins freely available, DeepMind catalyzed a global wave of community-driven research and collaboration. The DeepMind AlphaFold Protein Structure Database, developed in partnership with the European Molecular Biology Laboratory’s European Bioinformatics Institute (EMBL-EBI), now contains predicted structures for nearly all catalogued proteins, providing an unprecedented resource for life sciences.
In 2025, the open-source nature of AlphaFold continues to foster innovation. Researchers worldwide are leveraging the algorithm to accelerate drug discovery, understand disease mechanisms, and engineer novel proteins. The community has contributed improvements and extensions to the original code, such as adaptations for predicting protein complexes and protein-ligand interactions. Collaborative projects, often coordinated through open repositories and forums, have led to the development of user-friendly interfaces and integration with other bioinformatics tools, making AlphaFold accessible to a broader range of scientists, including those without deep expertise in machine learning.
Major scientific organizations, including National Institutes of Health (NIH) and RIKEN, have incorporated AlphaFold predictions into their research pipelines and databases. The EMBL-EBI continues to update and expand the AlphaFold Protein Structure Database, often in response to community feedback and emerging research needs. This collaborative ecosystem has enabled rapid responses to global health challenges, such as the identification of potential therapeutic targets for emerging infectious diseases.
Looking ahead, the open-source model is expected to remain central to AlphaFold’s evolution. Ongoing community efforts are focused on improving prediction accuracy for protein complexes, membrane proteins, and intrinsically disordered regions—areas where current models still face challenges. There is also a growing movement to integrate AlphaFold with other open-source platforms for genomics, cheminformatics, and systems biology, further enhancing its utility. The collaborative spirit fostered by AlphaFold’s open-source release is likely to drive continued breakthroughs in structural biology and related fields through 2025 and beyond.
Limitations, Challenges, and Ongoing Research
Since its landmark debut, the AlphaFold algorithm has revolutionized protein structure prediction, yet several limitations and challenges remain as of 2025. While DeepMind—the creator of AlphaFold—continues to refine the system, the scientific community is actively addressing its constraints and exploring new research directions.
One of the primary limitations of AlphaFold is its focus on predicting static, monomeric protein structures. Many biologically relevant proteins function as part of complexes or undergo significant conformational changes. AlphaFold’s predictions for protein-protein interactions, large assemblies, or intrinsically disordered regions are less reliable. Although the release of AlphaFold-Multimer in 2022 improved multimeric predictions, challenges persist in accurately modeling dynamic assemblies and transient interactions, which are crucial for understanding cellular mechanisms.
Another challenge is the algorithm’s reliance on high-quality sequence alignments and evolutionary data. For proteins with few homologs or those from poorly characterized organisms, AlphaFold’s accuracy diminishes. This limitation is particularly relevant for metagenomic proteins and novel sequences, which are increasingly important in biotechnology and environmental research.
AlphaFold also does not natively predict the effects of post-translational modifications, ligand binding, or the presence of cofactors, all of which can significantly alter protein structure and function. As a result, its utility in drug discovery and functional annotation is sometimes constrained, prompting ongoing research into integrating chemical and biophysical context into structure prediction.
The computational demands of AlphaFold, while reduced compared to traditional methods, remain significant for large-scale or high-throughput applications. Efforts are underway to optimize the algorithm for efficiency and to develop cloud-based platforms for broader accessibility. European Molecular Biology Laboratory’s European Bioinformatics Institute (EMBL-EBI) has partnered with DeepMind to provide the AlphaFold Protein Structure Database, which now contains hundreds of millions of predicted structures, but updating and expanding this resource remains a logistical and computational challenge.
Looking ahead, ongoing research is focused on several fronts: improving predictions for protein complexes and disordered regions, integrating experimental data (such as cryo-EM or NMR), and extending the algorithm to model protein-ligand and protein-nucleic acid interactions. The open-source release of AlphaFold’s code and models has catalyzed a global wave of innovation, with academic and industry groups worldwide contributing to its evolution. As these efforts mature, the next few years are expected to yield more accurate, context-aware, and functionally relevant protein structure predictions, further bridging the gap between computational models and biological reality.
Market and Public Interest: Growth and Forecasts
Since its public release, the AlphaFold algorithm has rapidly transformed the landscape of protein structure prediction, catalyzing significant market and public interest. Developed by DeepMind, a subsidiary of Alphabet Inc., AlphaFold’s open-source models and the subsequent expansion of the European Bioinformatics Institute (EMBL-EBI) AlphaFold Protein Structure Database have democratized access to high-accuracy protein structures. As of 2025, the database contains over 200 million predicted protein structures, covering nearly all catalogued proteins, and continues to expand in both scope and utility.
The market response has been robust, with biotechnology, pharmaceutical, and academic sectors integrating AlphaFold predictions into drug discovery, enzyme engineering, and disease research pipelines. Major pharmaceutical companies and research institutions are leveraging AlphaFold to accelerate target identification and reduce experimental costs, a trend expected to intensify through 2025 and beyond. The algorithm’s impact is also evident in the proliferation of startups and collaborative projects focused on protein design and synthetic biology, many of which cite AlphaFold as a foundational tool.
Forecasts for the next few years indicate sustained growth in both the adoption and application of AlphaFold and its derivatives. The DeepMind team, in collaboration with EMBL-EBI, has announced ongoing updates to the AlphaFold database, including improved accuracy for complex protein assemblies and integration with other omics data. These enhancements are expected to further broaden the algorithm’s utility in systems biology and personalized medicine.
Public interest remains high, as evidenced by the increasing number of citations in scientific literature and the widespread use of AlphaFold predictions in educational and citizen science initiatives. The open-access nature of the AlphaFold database has also spurred international collaborations, particularly in regions with limited experimental infrastructure, enabling a more equitable global research environment.
Looking ahead, the market for AI-driven protein structure prediction is projected to grow at a double-digit compound annual growth rate through the late 2020s, driven by ongoing advances in machine learning, cloud computing, and integration with laboratory automation. The continued commitment of organizations like DeepMind and EMBL-EBI to open science and resource sharing is likely to sustain both market momentum and public engagement, positioning AlphaFold as a central pillar in the future of computational biology.
Future Outlook: The Next Frontier in Computational Biology
The AlphaFold algorithm, developed by DeepMind, has rapidly transformed the landscape of computational biology since its landmark performance in the 2020 CASP14 competition. As of 2025, AlphaFold’s impact continues to expand, with its open-source models and the European Bioinformatics Institute (EMBL-EBI)’s AlphaFold Protein Structure Database now containing predictions for over 200 million proteins, covering nearly all catalogued sequences. This unprecedented resource is accelerating research in structural biology, drug discovery, and synthetic biology, enabling scientists to predict protein structures with remarkable accuracy and speed.
Looking ahead, the next few years are poised to see further advances in the AlphaFold algorithm and its applications. DeepMind and EMBL-EBI are actively collaborating to improve the accuracy of predictions for protein complexes and dynamic conformations, addressing current limitations in modeling protein-protein and protein-ligand interactions. These enhancements are critical for understanding cellular machinery and for the rational design of therapeutics, especially as the pharmaceutical industry increasingly integrates AI-driven structure prediction into early-stage drug development pipelines.
Moreover, the open availability of AlphaFold’s code and database is fostering a vibrant ecosystem of innovation. Research groups worldwide are building upon AlphaFold’s architecture to tackle related challenges, such as predicting the effects of genetic mutations on protein stability and function, and modeling intrinsically disordered proteins. Initiatives by organizations like National Institutes of Health and Kyoto University are leveraging AlphaFold’s predictions to annotate genomes and accelerate biomedical research, with a focus on rare diseases and emerging pathogens.
In the near future, integration of AlphaFold with other AI models and experimental data sources is expected to yield even more powerful hybrid approaches. For example, combining AlphaFold’s predictions with cryo-electron microscopy and mass spectrometry data could enable the reconstruction of entire cellular environments at atomic resolution. Additionally, the anticipated release of next-generation models—potentially incorporating advances in generative AI and unsupervised learning—may further improve the prediction of protein dynamics and interactions, opening new frontiers in systems biology and personalized medicine.
As computational power and algorithmic sophistication continue to grow, AlphaFold and its successors are set to play a central role in decoding the molecular basis of life, with profound implications for science, medicine, and biotechnology in the years ahead.
Sources & References
- DeepMind
- European Molecular Biology Laboratory’s European Bioinformatics Institute (EMBL-EBI)
- National Institutes of Health
- Royal Society of Chemistry
- Worldwide Protein Data Bank
- National Institutes of Health
- World Health Organization
- Research Collaboratory for Structural Bioinformatics (RCSB) Protein Data Bank
- World Health Organization (WHO)
- RIKEN
- European Bioinformatics Institute (EMBL-EBI)